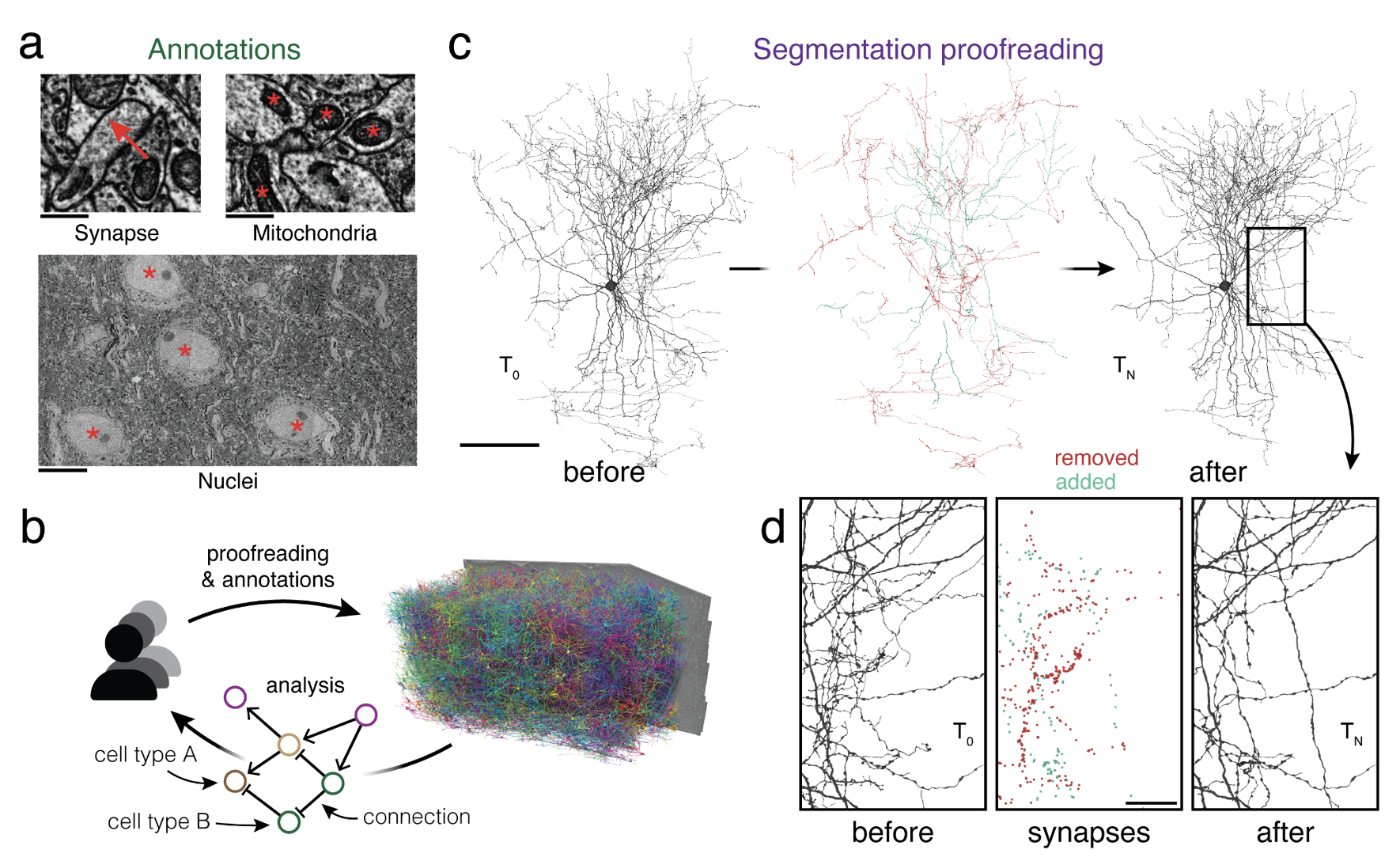
CAVE: Connectome Annotation Versioning Engine
bioRxiv, 2023.
Advances in Electron Microscopy, image segmentation and computational infrastructure have given rise to large-scale and richly annotated connectomic datasets which are increasingly shared across communities. To enable collaboration, users need to be able to concurrently create new annotations and correct errors in the automated segmentation by proofreading. In large datasets, every proofreading edit relabels cell identities of millions of voxels and thousands of annotations like synapses. For analysis, users require immediate and reproducible access to this constantly changing and expanding data landscape. Here, we present the Connectome Annotation Versioning Engine (CAVE), a computational infrastructure for immediate and reproducible connectome analysis in up-to petascale datasets (∼1mm3) while proofreading and annotating is ongoing. For segmentation, CAVE provides a distributed proofreading infrastructure for continuous versioning of large reconstructions. Annotations in CAVE are defined by locations such that they can be quickly assigned to the underlying segment which enables fast analysis queries of CAVE’s data for arbitrary time points. CAVE supports schematized, extensible annotations, so that researchers can readily design novel annotation types. CAVE is already used for many connectomics datasets, including the largest datasets available to date.
Acknowledgements
We thank John Wiggins, G. McGrath, and Dave Barlieb for computer system administration and M. Husseini for project administration. We thank Gregory Jefferis, Davi Bock, Eric Perlman, Philipp Schlegel, and Stephan Gerhard for providing feedback on the system design. We thank Gregory Jeffries, Phillip Schlegel, Brock Wester, Stephan Gerhard, Arie Matsliah, Brendan Celli, and Jake Reimer for building tools that leverage the CAVE infrastructure and providing consistent feedback on its performance and implementation. We thank Pedro Nunez Gomez for providing advice about deployment strategies. We would like to thank John Tuthill, Wei-Chung Allen Lee and the FANC community for their collaboration. We would like to thank Viren Jain, and Jeff Lichtman for collaboration on the H01 dataset. We would like to thank Stanley Heinze, Kevin Teodore for collaboration on their datasets. We would like to thank Zetta.ai for collaborations that use CAVE for further datasets. We would like to thank the FlyWire Consortium for collaboration on the FlyWire dataset. We thank the Allen Institute for Brain Science founder, P. G. Allen, for his vision, encouragement and support. Sebastian Seung acknowledges support from the National Institutes of Health (NIH) BRAIN Initiative RF1 MH129268, U24 NS126935, and RF1 MH123400, as well as assistance from Google. Forrest Collman, Nuno da Costa and Clay Reid acknowledge support from NIH RF1MH125932 and from NSF NeuroNex 2 award 2014862. Jakob Troidl and Hanspeter Pfister were supported by NSF grant NCS-FO-2124179. This work was supported by the Intelligence Advanced Research Projects Activity via Department of Interior/Interior Business Center contract numbers D16PC00004, D16PC0005, 2017-17032700004-005 and 2020-20081800401-023. The US Government is authorized to reproduce and distribute reprints for Governmental purposes notwithstanding any copyright annotation thereon. The views and conclusions contained herein are those of the authors and should not be interpreted as necessarily representing the official policies or endorsements, either expressed or implied, of Intelligence Advanced Research Projects Activity, ODNI, Department of Interior/Interior Business Center or the US Government.