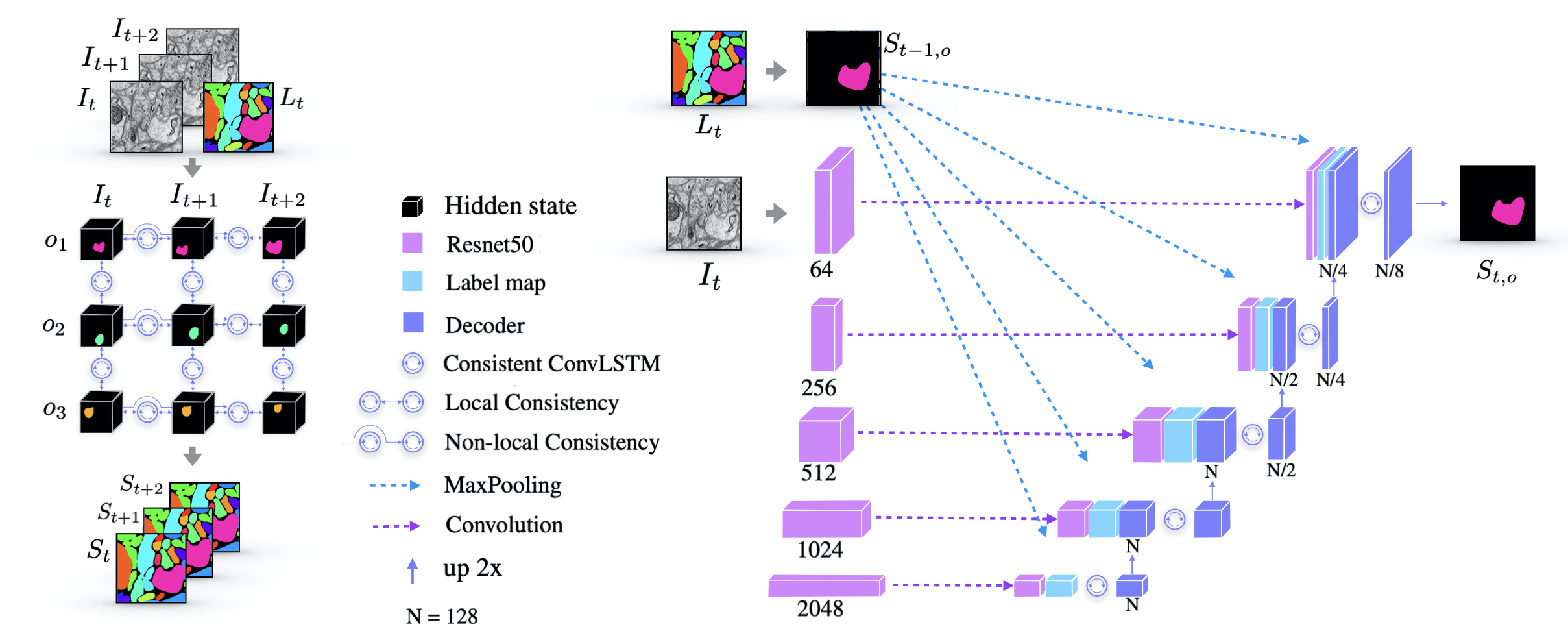
Consistent Recurrent Neural Networks for 3D Neuron Segmentation
To appear in the 2021 IEEE 18th International Symposium on Biomedical Imaging, 2021.
We present a recurrent network for the 3D reconstruction of neurons that sequentially generates binary masks for every object in an image with spatio-temporal consistency. Our network models consistency in two parts: (i) local, which allows exploring non-occluding and temporally-adjacent object relationships with bi-directional recurrence. (ii) non-local, which allows exploring long-range object relationships in the temporal domain with skip connections. Our proposed network is end-to-end trainable from an input image to a sequence of object masks, and, compared to methods relying on object boundaries, its output does not require post-processing. We evaluate our method on three benchmarks for neuron segmentation and achieved state-of-the-art performance on the SNEMI3D challenge.