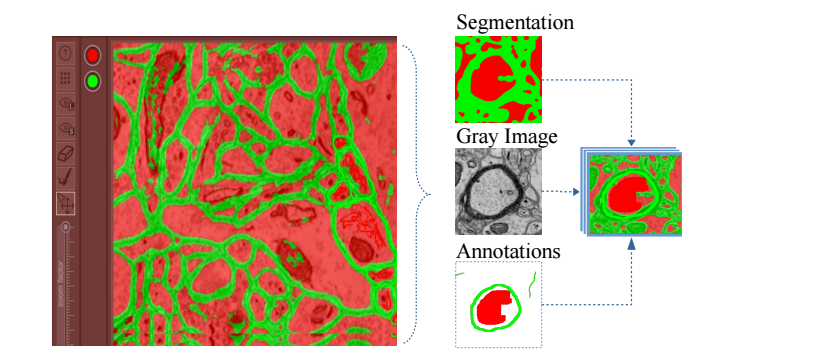
Icon: An Interactive Approach to Train Deep Neural Networks for Segmentation of Neuronal Structures
arXiv preprint arXiv:1610.09032, 2016.
We present an interactive approach to train a deep neural network pixel classifier for the segmentation of neuronal structures. An interactive training scheme reduces the extremely tedious manual annotation task that is typically required for deep networks to perform well on image segmentation problems. Our proposed method employs a feedback loop that captures sparse annotations using a graphical user interface, trains a deep neural network based on recent and past annotations, and displays the prediction output to users in almost real-time. Our implementation of the algorithm also allows multiple users to provide annotations in parallel and receive feedback from the same classifier. Quick feedback on classifier performance in an interactive setting enables users to identify and label examples that are more important than others for segmentation purposes. Our experiments show that an interactively-trained pixel classifier produces better region segmentation results on Electron Microscopy (EM) images than those generated by a network of the same architecture trained offline on exhaustive ground-truth labels.