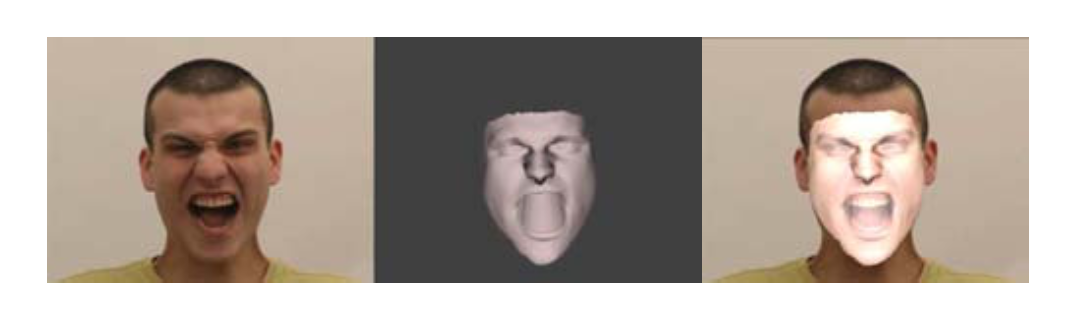
Multilinear Models for Face Synthesis
ACM: ACM SIGGRAPH 2004 Sketches, 2004.
Multilinear models offer a natural way of modeling heterogenous sources of variation. We are specifically interested in facial geometry variations due to identity and expression changes. In this setting, the multilinear model is able to capture idiosyncrasies such as style of smiling, i.e. the smile depends on the identity parameters. Two properties of multilinear models are of particular interest to animators: Separability – expression can be varied while identity stays constant, and vice versa; and Consistency – expression parameters encoding a smile for one person will encode a smile for every person spanned by the model, appropriate to their facial geometry and style of smiling. We introduce methods that make multilinear models a practical tool for animating faces, addressing two key obstacles. The key obstacle in constructing a multilinear model is the vast amount of data (in full correspondence) needed to account for every possible combination of attribute settings. The key problem in using a multilinear model is devising an intuitive control interface. For the data-acquisition problem, we show how to estimate a detailed multilinear model from an incomplete set of high-quality face scans. For the control problem, we show how to drive this model with a video performance, extracting identity, expression, and pose parameters.