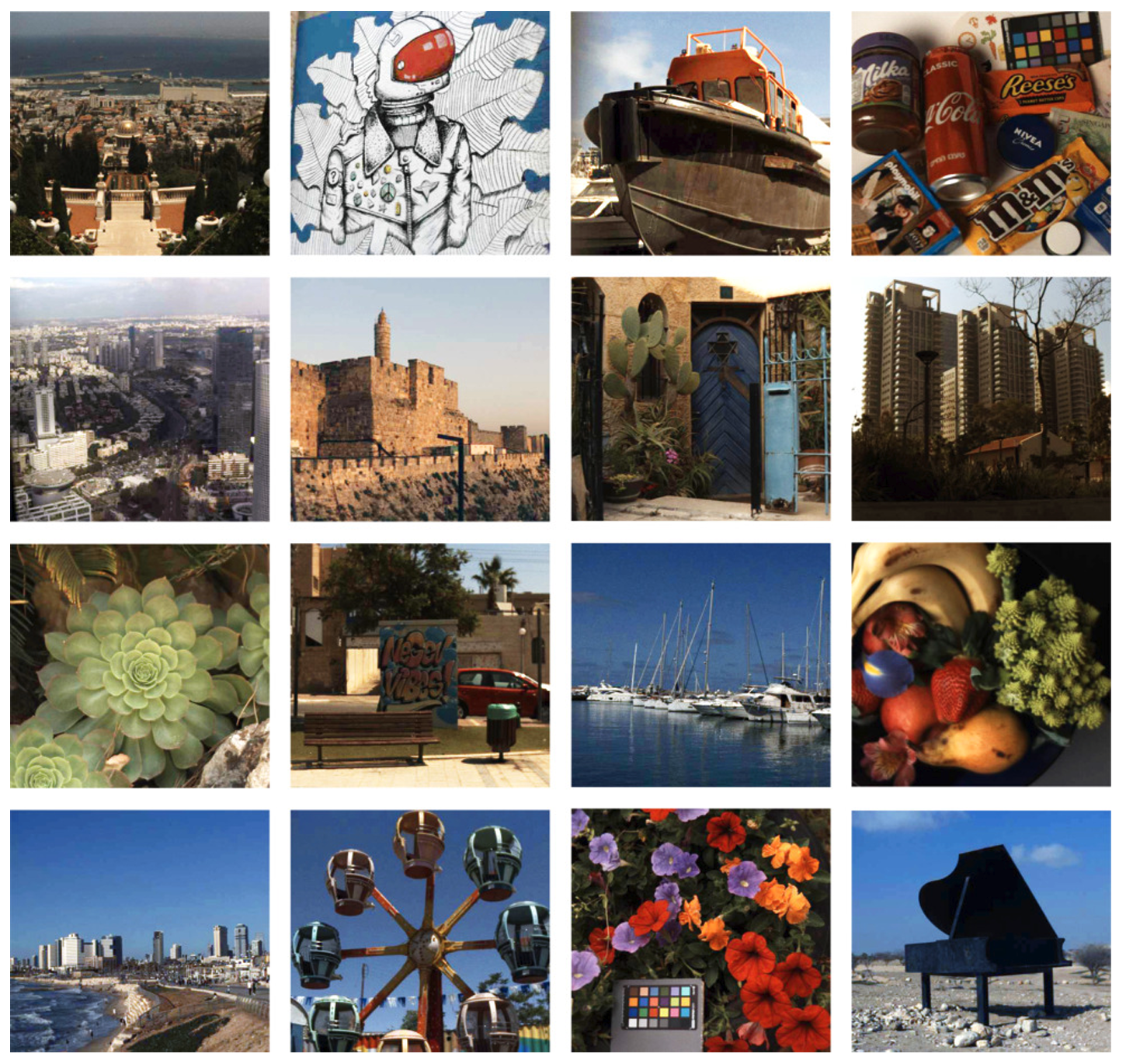
NTIRE 2022 Spectral Recovery Challenge and Data Set
Proceedings of the IEEE/CVF Conference on Computer Vision and Pattern Recognition (CVPR) Workshops, 2022.
This paper reviews the third biennial challenge on spectral reconstruction from RGB images, i.e., the recovery of whole-scene hyperspectral (HS) information from a 3-channel RGB image. This challenge presents the "ARAD_1K" data set: a new, larger-than-ever natural hyperspectral image data set containing 1,000 images. Challenge participants were required to recover hyperspectral information from synthetically generated JPEG-compressed RGB images simulating capture by a known calibrated camera, operating under partially known parameters, in a setting which includes acquisition noise. The challenge was attended by 241 teams, with 60 teams competing in the final testing phase, 12 of which provided detailed descriptions of their methodology which are included in this report. The performance of these submissions is reviewed and provided here as a gauge for the current state-of-the-art in spectral reconstruction from natural RGB images.
Acknowledgements
We thank the NTIRE 2022 sponsors: Huawei, Reality Labs, Bending Spoons, MediaTek, OPPO, Oddity, Voyage81, ETH Zurich (Computer Vision Lab) and University of Wurzburg (CAIDAS).