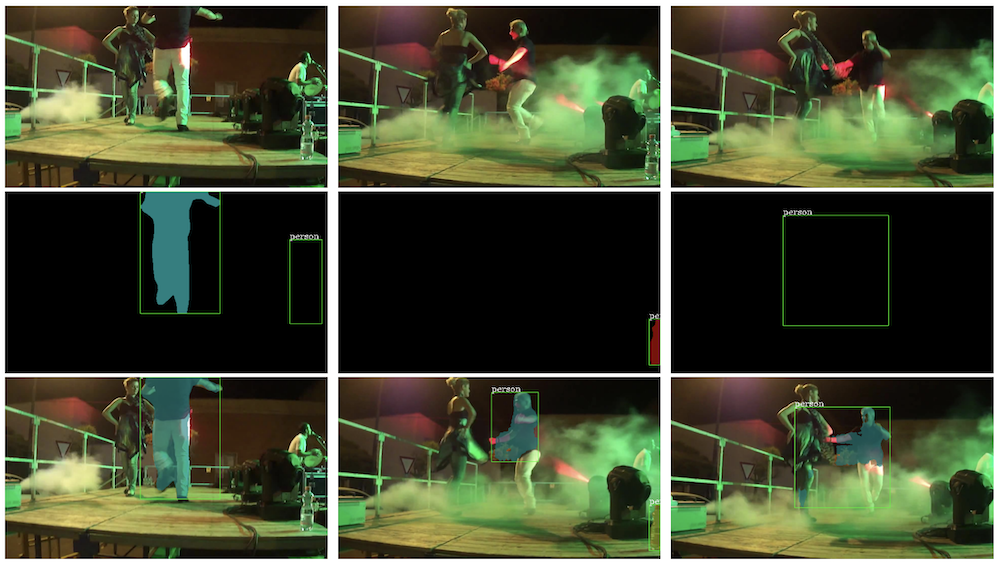
Object Propagation via Inter-Frame Attentions for Temporally Stable Video Instance Segmentation
arXiv preprint arXiv:2111.07529, 2021.
Video instance segmentation aims to detect, segment, and track objects in a video. Current approaches extend image-level segmentation algorithms to the temporal domain. However, this results in temporally inconsistent masks. In this work, we identify the mask quality due to temporal stability as a performance bottleneck. Motivated by this, we propose a video instance segmentation method that alleviates the problem due to missing detections. Since this cannot be solved simply using spatial information, we leverage temporal context using inter-frame attentions. This allows our network to refocus on missing objects using box predictions from the neighbouring frame, thereby overcoming missing detections. Our method significantly outperforms previous state-of-the-art algorithms using the Mask R-CNN backbone, by achieving 36.0% mAP on the YouTube-VIS benchmark. Additionally, our method is completely online and requires no future frames.