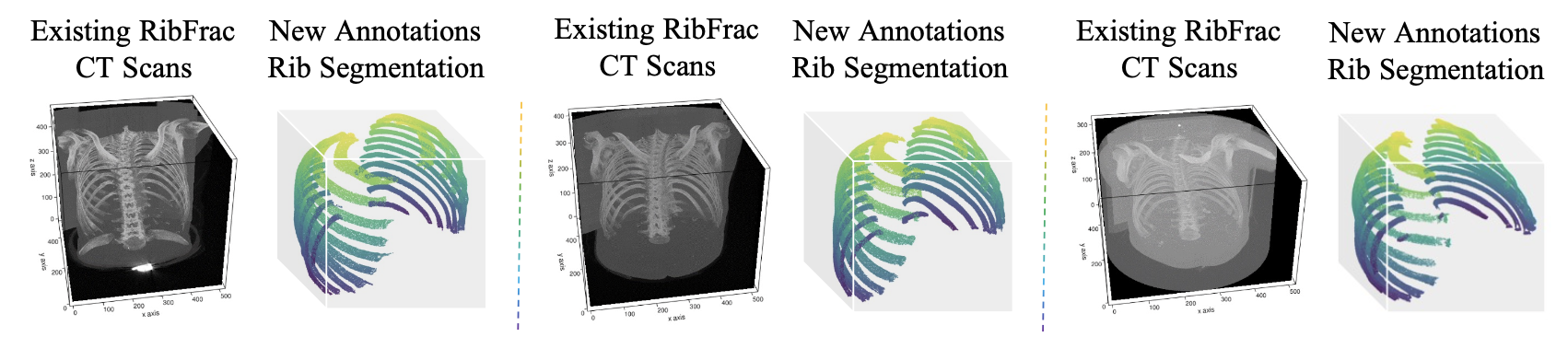
RibSeg Dataset and Strong Point Cloud Baselines for Rib Segmentation from CT Scans
(MICCAI), 2021.
Manual rib inspections in computed tomography (CT) scans are clinically critical but labor-intensive, as 24 ribs are typically elon- gated and oblique in 3D volumes. Automatic rib segmentation methods can speed up the process through rib measurement and visualization. However, prior arts mostly use in-house labeled datasets that are pub- licly unavailable and work on dense 3D volumes that are computationally inefficient. To address these issues, we develop a labeled rib segmentation benchmark, named RibSeg, including 490 CT scans (11,719 individual ribs) from a public dataset. For ground truth generation, we used exist- ing morphology-based algorithms and manually refined its results. Then, considering the sparsity of ribs in 3D volumes, we thresholded and sam- pled sparse voxels from the input and designed a point cloud-based base- line method for rib segmentation. The proposed method achieves state- of-the-art segmentation performance (Dice ≈ 95%) with significant effi- ciency (10 ∼ 40× faster than prior arts). The RibSeg dataset, code, and model in PyTorch are available at https://github.com/M3DV/RibSeg.