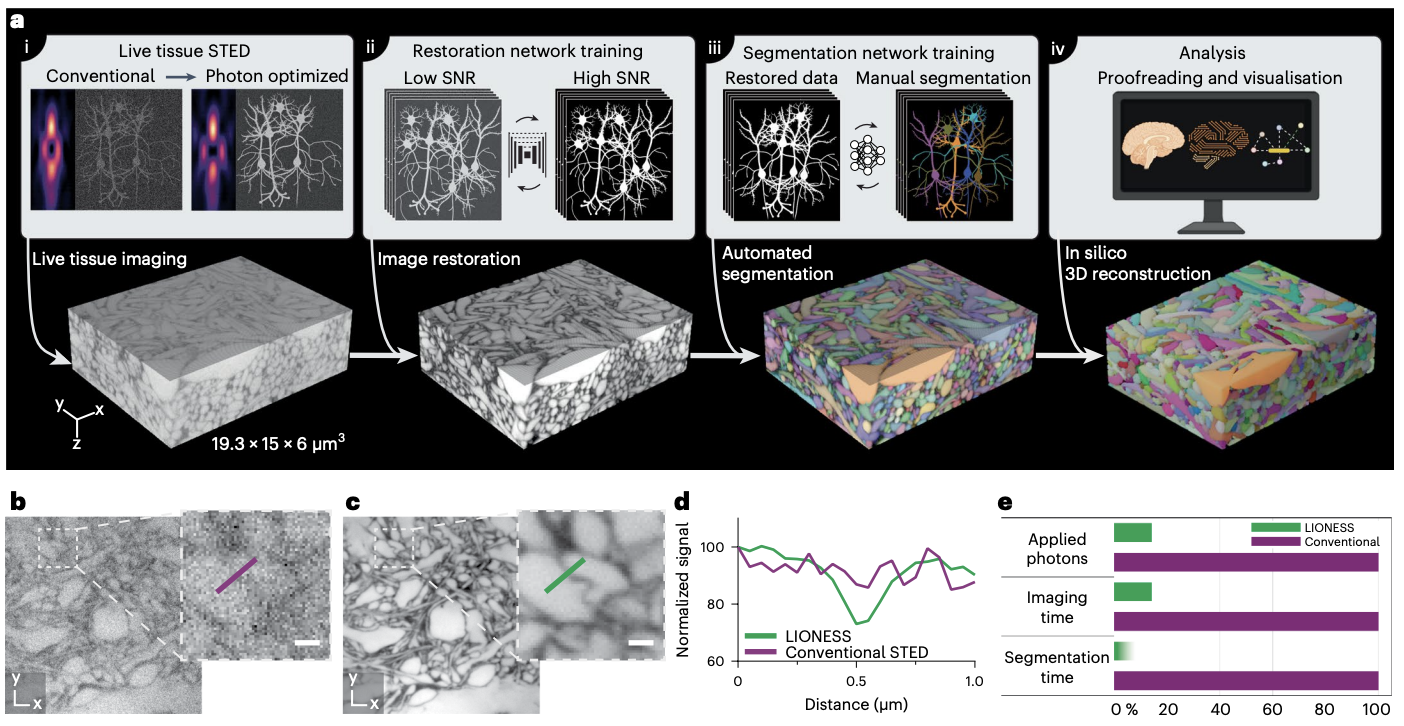
Dense 4D nanoscale reconstruction of living brain tissue
Nature Methods, 2023.
Three-dimensional (3D) reconstruction of living brain tissue down to an individual synapse level would create opportunities for decoding the dynamics and structure–function relationships of the brain’s complex and dense information processing network; however, this has been hindered by insufficient 3D resolution, inadequate signal-to-noise ratio and prohibitive light burden in optical imaging, whereas electron microscopy is inherently static. Here we solved these challenges by developing an integrated optical/machine-learning technology, LIONESS (live information-optimized nanoscopy enabling saturated segmentation). This leverages optical modifications to stimulated emission depletion microscopy in comprehensively, extracellularly labeled tissue and previous information on sample structure via machine learning to simultaneously achieve isotropic super-resolution, high signal-to-noise ratio and compatibility with living tissue. This allows dense deep-learning-based instance segmentation and 3D reconstruction at a synapse level, incorporating molecular, activity and morphodynamic information. LIONESS opens up avenues for studying the dynamic functional (nano-)architecture of living brain tissue.
Acknowledgements
We thank J. Vorlaufer, N. Agudelo and A. Wartak for microscope maintenance and troubleshooting, C. Kreuzinger and A. Freeman for technical assistance, M. Šuplata for hardware control support and M. Cunha dos Santos for initial exploration of software. We thank P. Henderson for advice on deep-learning training and M. Sixt, S. Boyd and T. Weiss for discussions and critical reading of the manuscript. L. Lavis (Janelia Research Campus) generously provided the JF585-HaloTag ligand. We acknowledge expert support by IST Austria’s scientific computing, imaging and optics, preclinical, library and laboratory support facilities and by the Miba machine shop. We gratefully acknowledge funding by the following sources: Austrian Science Fund (F.W.F.) grant no. I3600-B27 (J.G.D.), grant no. DK W1232 (J.G.D. and J.M.M.) and grant no. Z 312-B27, Wittgenstein award (P.J.); the Gesellschaft für Forschungsförderung NÖ grant no. LSC18-022 (J.G.D.); an ISTA Interdisciplinary project grant (J.G.D. and B.B.); the European Union’s Horizon 2020 research and innovation programme, Marie-Skłodowska Curie grant 665385 (J.M.M. and J.L.); the European Union’s Horizon 2020 research and innovation programme, European Research Council grant no. 715767, MATERIALIZABLE (B.B.); grant no. 715508, REVERSEAUTISM (G.N.); grant no. 695568, SYNNOVATE (S.G.N.G.); and grant no. 692692, GIANTSYN (P.J.); the Simons Foundation Autism Research Initiative grant no. 529085 (S.G.N.G.); the Wellcome Trust Technology Development grant no. 202932 (S.G.N.G.); the Marie Skłodowska-Curie Actions Individual Fellowship no. 101026635 under the EU Horizon 2020 program (J.F.W.); the Human Frontier Science Program postdoctoral fellowship LT000557/2018 (W.J.); and the National Science Foundation grant no. IIS-1835231 (H.P.) and NCS-FO-2124179 (H.P.). The funders had no role in study design, data collection and analysis, decision to publish or preparation of the manuscript.