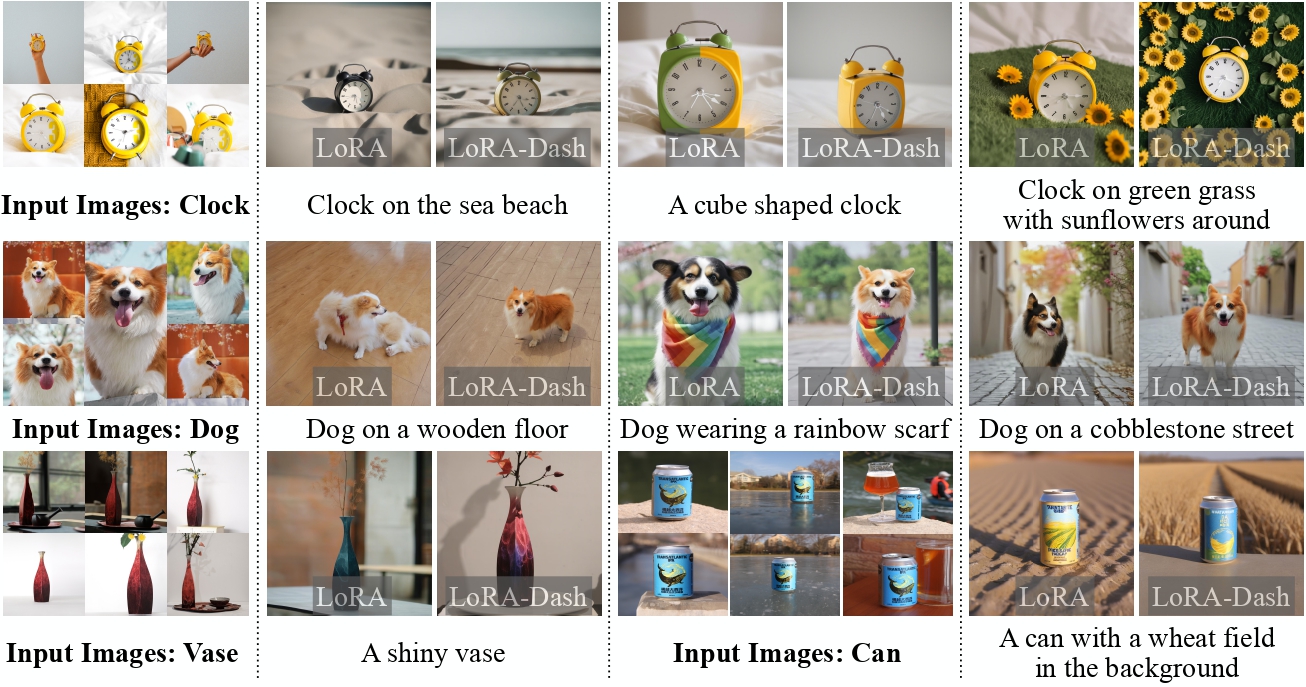
Unleashing the Power of Task-Specific Directions in Parameter Efficient Fine-tuning
International Conference on Learning Representations(ICLR), 2025.
Large language models demonstrate impressive performance on downstream tasks, yet requiring extensive resource consumption when fully fine-tuning all parameters. To mitigate this, Parameter Efficient Fine-Tuning (PEFT) strategies, such as LoRA, have been developed. In this paper, we delve into the concept of task-specific directions (TSDs)-critical for transitioning large models from pretrained states to task-specific enhancements in PEFT. We propose a framework to clearly define these directions and explore their properties, and practical utilization challenges. We then introduce a novel approach, LoRA-Dash, which aims to maximize the impact of TSDs during the fine-tuning process, thereby enhancing model performance on targeted tasks. Extensive experiments have conclusively demonstrated the effectiveness of LoRA-Dash, and in-depth analyses further reveal the underlying mechanisms of LoRA-Dash. The code is available at this https URL.