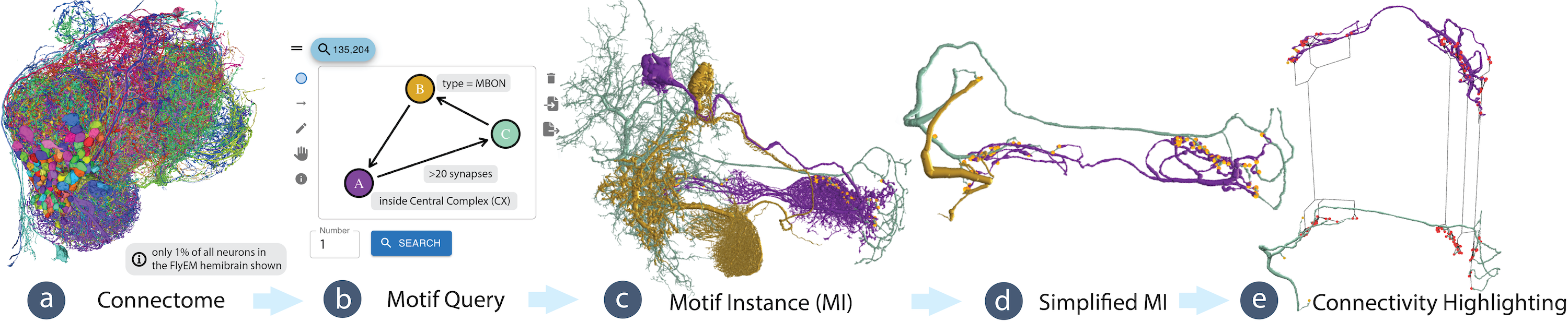
Vimo: Visual Analysis of Neuronal Connectivity Motifs
IEEE Transactions on Visualization and Computer Graphics (IEEE VIS), 2023.
Recent advances in high-resolution connectomics provide researchers with access to accurate petascale reconstructions of neuronal circuits and brain networks for the first time. Neuroscientists are analyzing these networks to better understand information processing in the brain. In particular, scientists are interested in identifying specific small network motifs, i.e., repeating subgraphs of the larger brain network that are believed to be neuronal building blocks. Although such motifs are typically small (e.g., 2 - 6 neurons), the vast data sizes and intricate data complexity present significant challenges to the search and analysis process. To analyze these motifs, it is crucial to review instances of a motif in the brain network and then map the graph structure to detailed 3D reconstructions of the involved neurons and synapses. We present Vimo, an interactive visual approach to analyze neuronal motifs and motif chains in large brain networks. Experts can sketch network motifs intuitively in a visual interface and specify structural properties of the involved neurons and synapses to query large connectomics datasets. Motif instances (MIs) can be explored in high-resolution 3D renderings. To simplify the analysis of MIs, we designed a continuous focus&context metaphor inspired by visual abstractions. This allows users to transition from a highly-detailed rendering of the anatomical structure to views that emphasize the underlying motif structure and synaptic connectivity. Furthermore, Vimo supports the identification of motif chains where a motif is used repeatedly (e.g., 2 - 4 times) to form a larger network structure. We evaluate Vimo in a user study with seven domain experts and an in-depth case study on motifs in a large connectome of the fruit fly, including more than 21,000 neurons and 20 million synapses. We find that Vimo enables hypothesis generation and confirmation through fast analysis iterations and connectivity highlighting.
Acknowledgements
This work was supported by NSF awards IIS-1901030, NSF-IIS2239688, NCS-FO-2124179, and R24MH114785. We gratefully acknowledge internal financial support from the Johns Hopkins University Applied Physics Laboratory’s Independent Research & Development (IR&D) Program for funding portions of this work. We also thank the participants of our user study and the anonymous reviewers for their valuable feedback.