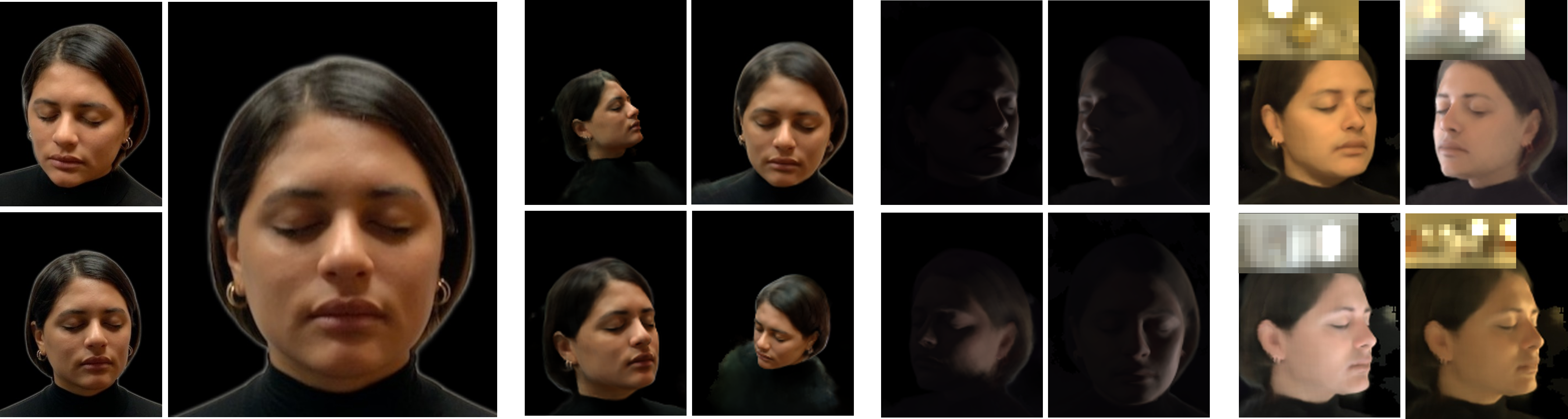
VoRF: Volumetric Relightable Faces
(BMVC), 2022.
3D generative models of objects enable photorealistic image synthesis with 3D control. Existing methods model the scene as a global scene representation, ignoring the compositional aspect of the scene. Compositional reasoning can enable a wide variety of editing applications, in addition to enabling generalizable 3D reasoning. In this paper, we present a compositional generative model, where each semantic part of the object is represented as an independent 3D representation learnt from only in-the-wild 2D data. We start with a global generative model (GAN) and learn to decompose it into different semantic parts using supervision from 2D segmentation masks. We then learn to composite independently sampled parts in order to create coherent global scenes. Different parts can be independently sampled, while keeping rest of the object fixed. We evaluate our method on a wide variety of objects and parts, and demonstrate editing applications.
Acknowledgements
This work was supported by the ERC Consolidator Grant 4DReply (770784).