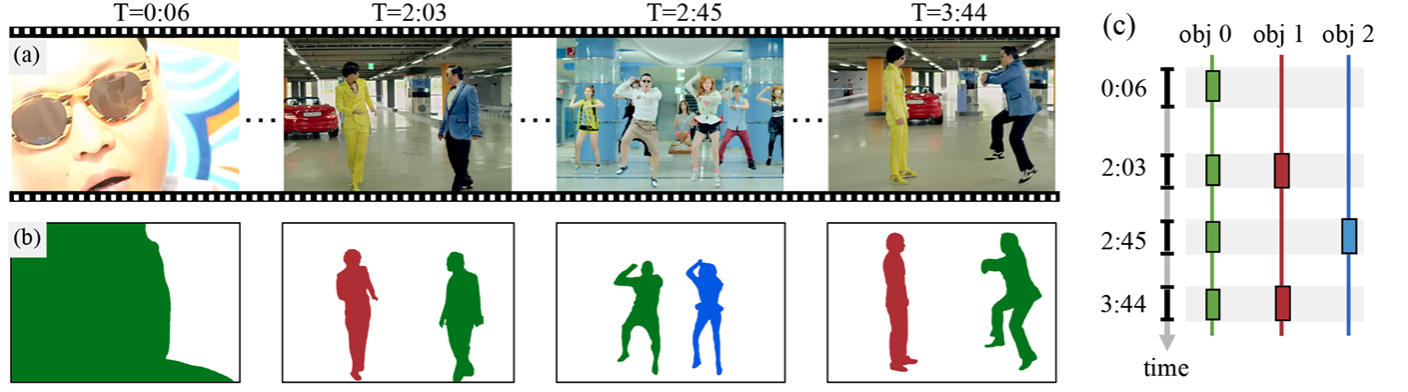
YouMVOS: An Actor-Centric Multi-Shot Video Object Segmentation Dataset
Proceedings of the IEEE/CVF Conference on Computer Vision and Pattern Recognition (CVPR), 2022.
Many video understanding tasks require analyzing multi-shot videos, but existing datasets for video object segmentation (VOS) only consider single-shot videos. To address this challenge, we collected a new dataset---YouMVOS---of 200 popular YouTube videos spanning ten genres, where each video is on average five minutes long and with 75 shots. We selected recurring actors and annotated 431K segmentation masks at a frame rate of six, exceeding previous datasets in average video duration, object variation, and narrative structure complexity. We incorporated good practices of model architecture design, memory management, and multi-shot tracking into an existing video segmentation method to build competitive baseline methods. Through error analysis, we found that these baselines still fail to cope with cross-shot appearance variation on our YouMVOS dataset. Thus, our dataset poses new challenges in multi-shot segmentation towards better video analysis. Data, code, and pre-trained models are available.
Acknowledgements
This work has been supported by NSF grants NCS-FO2124179, NIH grant R01HD104969, UKRI grant Turing AI Fellowship EP/W002981/1, andE PSRC/MURI grant EP/N019474/1. We also thank the Royal Academy of Engineering and Five AI.