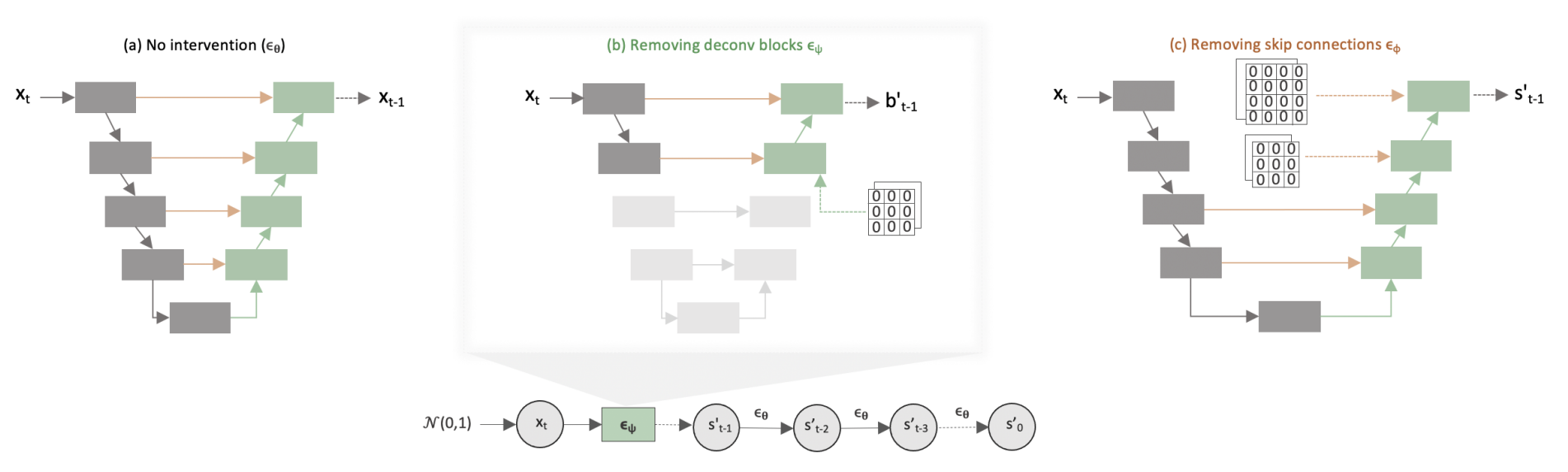
Unraveling the Temporal Dynamics of the Unet in Diffusion Models
Deployable AI @ AAAI 2024, 2023.
Diffusion models have garnered significant attention since they can effectively learn complex multivariate Gaussian distributions, resulting in diverse, high-quality outcomes. They introduce Gaussian noise into training data and reconstruct the original data iteratively. Central to this iterative process is a single Unet, adapting across time steps to facilitate generation. Recent work revealed the presence of composition and denoising phases in this generation process, raising questions about the Unets’ varying roles. Our study dives into the dynamic behavior of Unets within denoising diffusion probabilistic models (DDPM), focusing on (de)convolutional blocks and skip connections across time steps. We propose an analytical method to systematically assess the impact of time steps and core Unet components on the final output. This method eliminates components to study causal relations and investigate their influence on output changes. The main purpose is to understand the temporal dynamics and identify potential shortcuts during inference. Our findings provide valuable insights into the various generation phases during inference and shed light on the Unets’ usage patterns across these phases. Leveraging these insights, we identify redundancies in GLIDE (an improved DDPM) and improve inference time by ∼27% with minimal degradation in output quality. Our ultimate goal is to guide more informed optimization strategies for inference and influence new model designs.