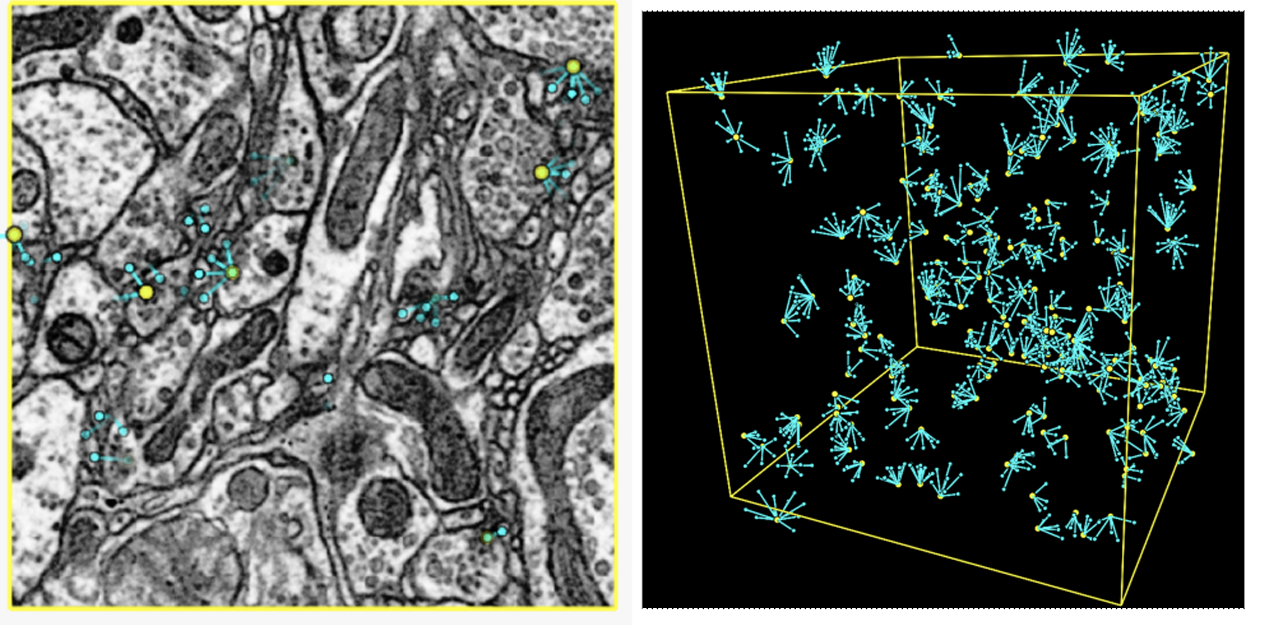
An Out-of-Domain Synapse Detection Challenge for Microwasp Brain Connectomes
(Challenge at IEEE ISBI), 2023.
The size of image stacks in connectomics studies now reaches the terabyte and often petabyte scales with a great diversity of appearance across brain regions and samples. However, manual annotation of neural structures, e.g., synapses, is time-consuming, which leads to limited training data often smaller than 0.001% of the test data in size. Domain adaptation and generalization approaches were proposed to address similar issues for natural images, which were less evaluated on connectomics data due to a lack of out-of-domain benchmarks. This challenge aims to push the boundary of out-of-domain generalization methods for large-scale connectomics applications. To facilitate this challenge, we annotated 14 image chunks from a biologically diverse set of Megaphragma viggianii brain regions in three whole-brain datasets. Successful algorithms that emerge from our challenge could potentially revolutionize real-world connectomics research and further efforts that aim to unravel the complexity of brain structure and function.
Acknowledgements
We appreciate the hard work of ground truth annotators: Diane Nguyen, Chi-Yip Ho, Aya Shinomiya, Sonia Villani, and Myisha Thasin. The images were acquired and aligned by Song Pang and C. Shan Xu in the lab of Harald Hess at Janelia Research Campus. This is supported by the Howard Hughes Medical Institute. The research at Flatiron Institute and the competition award are funded by Simons Foundation. The sample preparation is supported by Russian Science Foundation (project no. 22-1400028 to AP). This work was partially supported by NSF grant NCS-FO2124179.